Real Time Applications of Big Data Analytics
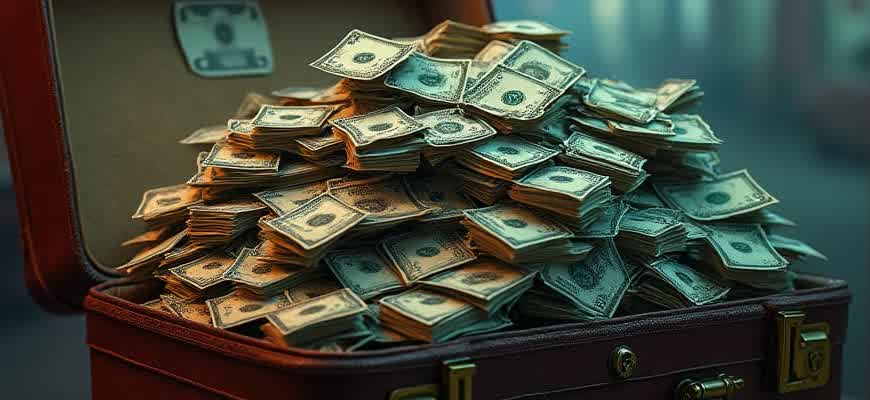
Big data analytics has transformed various industries by providing real-time insights and enabling faster decision-making. One of the most significant advantages of big data is its ability to process large volumes of data as it is generated, offering immediate responses for critical operations. Below are some prominent applications:
- Healthcare: Real-time patient monitoring, predictive analytics for treatment outcomes, and personalized medicine.
- Finance: Fraud detection, algorithmic trading, and real-time risk assessment.
- Retail: Customer behavior analysis, inventory management, and dynamic pricing strategies.
"The value of real-time analytics lies in its ability to provide immediate actionable insights, transforming how industries respond to challenges and opportunities."
These applications rely on advanced technologies such as machine learning, IoT devices, and cloud computing. A key component of success in real-time analytics is the integration of structured and unstructured data sources. The table below highlights some of the technologies driving these changes:
Technology | Application |
---|---|
IoT | Real-time data collection and monitoring from connected devices. |
Cloud Computing | Scalable processing power to handle large data streams in real-time. |
Machine Learning | Predictive models that learn from data in real-time to provide instant insights. |
Optimizing Supply Chain Management with Real-Time Data
Supply chain management has undergone a dramatic transformation with the integration of real-time data analytics. With advanced technologies like IoT sensors, GPS tracking, and RFID, companies can now monitor inventory levels, shipments, and production processes in real-time. This level of visibility allows businesses to respond promptly to disruptions, adjust their operations dynamically, and maintain better control over their entire supply chain.
Real-time analytics enable more accurate decision-making, improved operational efficiency, and better customer satisfaction. By leveraging this data, organizations can predict potential issues, optimize routes, and enhance forecasting, ultimately reducing operational costs and improving product availability. This capability is crucial for businesses that operate in competitive markets with fluctuating demand and unpredictable conditions.
Key Benefits of Real-Time Supply Chain Analytics
- Improved Decision-Making: Immediate access to data allows managers to make informed decisions quickly and accurately.
- Cost Reduction: Real-time insights help identify inefficiencies, allowing companies to optimize resources and minimize waste.
- Enhanced Visibility: Tracking goods and shipments provides transparency throughout the entire supply chain, preventing delays.
- Proactive Risk Management: Real-time alerts help businesses anticipate and mitigate potential disruptions.
Real-Time Data Applications in Supply Chain
- Inventory Management: Monitoring stock levels and automatically reordering when items run low ensures that warehouses are stocked efficiently without overstocking.
- Route Optimization: By using real-time traffic and weather data, businesses can optimize delivery routes to minimize delays and fuel costs.
- Supplier Coordination: Real-time tracking of shipments and deliveries allows for better coordination between suppliers and manufacturers, reducing lead times.
Example: Real-Time Data Integration in Supply Chain
Stage | Real-Time Data Used | Impact |
---|---|---|
Inventory Monitoring | Automated alerts based on stock levels | Prevents stockouts and overstocking |
Logistics and Delivery | Real-time GPS tracking and traffic data | Optimizes delivery routes, reduces delays |
Supplier Communication | Real-time shipment updates | Improves lead time accuracy and collaboration |
Real-time data analytics not only optimize the internal processes of the supply chain but also strengthen the relationships between stakeholders by providing a seamless flow of information.
Enhancing Customer Experience Through Real-Time Personalization
In today's highly competitive market, businesses are increasingly leveraging big data analytics to provide a tailored experience to their customers. Real-time data analysis allows companies to gain deep insights into consumer behavior, preferences, and actions. This immediate access to information enables personalized experiences that are more relevant and engaging, fostering stronger customer loyalty and satisfaction.
Real-time personalization not only enhances the user experience but also drives immediate actions. By analyzing customer data as it is generated, companies can predict needs, offer timely recommendations, and adapt their services based on the unique behavior of each user. This approach helps businesses deliver highly relevant content or product suggestions in a dynamic and immediate manner.
Key Strategies for Real-Time Personalization
- Customer Segmentation: Dividing customers into groups based on behavior patterns or preferences helps deliver personalized messages or offers.
- Product Recommendations: Analyzing browsing history or past purchases to suggest products or services customers are most likely to engage with.
- Location-Based Personalization: Using geolocation data to send location-specific offers, promotions, or services in real time.
"The ability to deliver personalized content in real time increases engagement and significantly boosts conversion rates."
Example of Real-Time Personalization in Action
Strategy | Result |
---|---|
Dynamic Website Content | Increased average time spent on site by 40% |
Personalized Email Campaigns | Boosted open rates by 25% |
Real-Time Push Notifications | Enhanced user interaction by 30% |
Real-time personalization offers businesses a competitive edge by tailoring interactions based on the evolving needs and preferences of each customer. By continuously adapting content and offers to individual behaviors, companies can significantly enhance their customer experience and improve overall satisfaction.
Real-Time Fraud Detection in Financial Transactions
In the modern financial ecosystem, the ability to detect fraudulent activities as they happen is critical for preventing significant monetary losses. The integration of big data analytics allows for rapid detection of anomalous patterns and suspicious behavior in financial transactions. By processing vast amounts of transaction data in real time, financial institutions can identify fraudulent activities within seconds, providing immediate responses to mitigate risk.
Big data techniques leverage advanced machine learning algorithms and statistical models to analyze user behavior, transaction histories, and network patterns. This allows for the identification of deviations from normal transaction patterns, such as unusual spending locations, times, or amounts. Once a potential fraud is detected, the system can immediately flag the transaction and even block further actions until verification is complete.
Key Components of Real-Time Fraud Detection
- Data Integration: The system aggregates data from multiple sources, including transaction logs, user accounts, and device information, to form a comprehensive view of the transaction.
- Behavioral Analysis: Analyzing past behavior patterns of users helps create baseline profiles that can quickly detect anomalies in real-time.
- Machine Learning Models: Supervised and unsupervised learning techniques help in classifying transactions as either legitimate or suspicious based on historical data and emerging trends.
Process Flow of Fraud Detection
- Transaction data is collected in real-time from various channels (e.g., mobile apps, websites, ATM withdrawals).
- The system analyzes the data against predefined models and user behavior profiles.
- Once a transaction is flagged as potentially fraudulent, it is immediately reviewed, either by automated systems or human analysts.
- The transaction is either blocked or processed based on the review outcome.
Challenges in Real-Time Fraud Detection
Challenge | Impact | Solution |
---|---|---|
High False Positive Rate | Increased operational costs and customer inconvenience. | Refining machine learning models to improve accuracy. |
Volume of Data | Difficulty in processing vast amounts of data in real time. | Using distributed computing and parallel processing frameworks. |
Adaptive Fraud Techniques | Fraudsters changing tactics to bypass detection. | Continuous training and updating of fraud detection algorithms. |
Real-time fraud detection systems are essential in maintaining financial security, as they enable quick identification and mitigation of potentially fraudulent activities.
Leveraging Big Data for Real-Time Healthcare Monitoring
The integration of real-time data analytics into healthcare has opened up new avenues for improving patient care. By utilizing large-scale data collected from various sources such as wearable devices, electronic health records (EHR), and medical imaging systems, healthcare providers can make informed decisions on the spot. This capability not only enhances the accuracy of diagnoses but also aids in timely interventions, significantly improving patient outcomes. With the increasing amount of health-related data generated every second, the role of big data analytics in real-time healthcare monitoring becomes even more critical.
Big data analytics facilitates the continuous tracking of patient health metrics, enabling proactive management of chronic conditions and detecting potential health risks before they escalate. The ability to process vast amounts of data in real time allows healthcare professionals to make rapid, data-driven decisions, enhancing the effectiveness of treatment plans and minimizing unnecessary hospital visits.
Key Benefits of Real-Time Healthcare Monitoring Using Big Data
- Early Detection of Health Issues: Real-time analysis of patient data enables the identification of abnormal patterns in health indicators, leading to early diagnosis of conditions such as heart disease, diabetes, and hypertension.
- Personalized Treatment Plans: By continuously monitoring patients, healthcare providers can tailor treatments to individual needs, improving overall effectiveness and reducing side effects.
- Improved Resource Allocation: Big data analytics helps in managing hospital resources more efficiently by predicting patient needs, optimizing bed availability, and reducing wait times.
Applications of Real-Time Data in Healthcare Monitoring
- Wearable Health Devices: Devices like smartwatches and fitness trackers continuously monitor vital signs such as heart rate, oxygen levels, and sleep patterns.
- Remote Patient Monitoring (RPM): Remote monitoring solutions enable healthcare providers to keep track of patients' health without the need for in-person visits, especially for those with chronic conditions.
- Emergency Alerts: In critical situations, real-time analytics can trigger automated alerts for medical staff, allowing for quick response to emergencies like cardiac arrest or respiratory failure.
"By integrating real-time monitoring and predictive analytics, healthcare professionals can not only treat existing conditions but also prevent future health crises from occurring."
Examples of Real-Time Monitoring Technologies
Technology | Functionality | Impact |
---|---|---|
Smart Wearables | Track vitals such as heart rate, steps, and sleep patterns in real-time | Provide continuous health monitoring and data-driven insights for early interventions |
Telemedicine Platforms | Enable remote consultations and real-time health data sharing between patients and providers | Facilitate timely diagnosis and reduce the need for in-person visits |
AI-Powered Diagnostics | Analyze medical data (e.g., lab results, imaging) to detect anomalies and suggest diagnoses | Enhance accuracy in diagnosis and speed up treatment decision-making |
Real-Time Traffic Monitoring for Smart City Innovations
Modern cities are leveraging big data to enhance urban mobility through real-time traffic analysis, providing dynamic solutions for congestion, safety, and overall efficiency. These solutions use data from sensors, cameras, and GPS devices to collect traffic flow information in real-time. This data is then analyzed and applied to optimize traffic signals, improve public transport routes, and inform drivers about road conditions instantly.
Through the application of real-time analytics, urban planners and city managers can identify issues like traffic bottlenecks, accident-prone areas, and irregularities in traffic patterns. This allows for proactive measures, such as adjusting signal timings or directing traffic away from heavily congested zones. The technology helps create smoother and more responsive city infrastructure, contributing to better quality of life for residents.
Key Components of Real-Time Traffic Systems
- Traffic Flow Monitoring: Continuous data collection through sensors embedded in roads or attached to vehicles.
- Signal Optimization: Dynamic adjustment of traffic lights based on real-time traffic data.
- Incident Detection: Early detection of accidents or breakdowns, allowing for quick response and rerouting.
- Public Transport Integration: Real-time updates on traffic conditions that can adjust public transport routes and schedules.
Benefits of Real-Time Traffic Analytics
"Smart traffic management enables cities to become more adaptive, reducing congestion and improving commuter experiences."
- Improved Traffic Flow: Efficient signal management reduces unnecessary stops and delays.
- Accident Reduction: Fast detection and rerouting minimize the impact of accidents on traffic.
- Environmental Benefits: Less idle time in traffic reduces fuel consumption and emissions.
- Enhanced Public Safety: Timely responses to accidents and hazardous conditions protect both drivers and pedestrians.
Example: Traffic Data in Action
Location | Traffic Condition | Action Taken |
---|---|---|
Downtown Intersection | Heavy Traffic | Adjusted Signal Timing |
Bridge Approach | Accident Detected | Rerouted Traffic |
Main Avenue | Clear | Normal Flow |
Real-Time Data-Driven Marketing Strategies for E-commerce
In today's competitive e-commerce landscape, leveraging real-time data is essential for creating personalized and effective marketing campaigns. Real-time analytics enable businesses to respond instantly to customer behaviors, market trends, and inventory changes. By using big data tools to track user interactions on websites, mobile apps, and social media, companies can implement targeted strategies that drive engagement and sales.
Effective data-driven marketing strategies can significantly improve customer retention, boost conversion rates, and enhance brand loyalty. These strategies rely on insights gained from monitoring and analyzing data in real-time, which allows for agile decision-making and timely adjustments to marketing campaigns.
Key Real-Time Marketing Techniques
- Behavioral Targeting: By tracking user behavior, such as browsing patterns and purchase history, businesses can serve personalized ads and recommendations in real-time.
- Dynamic Pricing: Real-time analysis of market conditions and customer demand enables businesses to adjust product prices instantly, offering competitive rates.
- Instant Offers: Sending personalized discounts or promotions based on a customer's current activity or location can lead to immediate conversions.
Benefits of Real-Time Marketing for E-Commerce
- Improved Customer Experience: Providing personalized content and offers enhances the user experience, making customers feel more valued and understood.
- Increased Conversion Rates: Real-time adjustments to marketing campaigns based on customer actions can lead to higher conversion rates.
- Faster Response to Market Changes: The ability to analyze and respond to data quickly helps businesses stay ahead of competitors.
Example of Real-Time Data in Marketing
Action | Real-Time Response | Result |
---|---|---|
Customer browses a product page | Display personalized product recommendations | Increased chances of cross-selling and upselling |
Customer adds items to the cart but doesn't complete the purchase | Send a targeted email with a limited-time discount | Increased cart abandonment recovery |
"Real-time data allows businesses to act immediately on new insights, transforming customer interactions into personalized experiences that drive higher engagement and revenue."
Improving Operational Decision-Making with Live Data Streams
In modern industries, real-time data analysis is essential for making informed operational decisions. The ability to process data as it flows through systems allows organizations to quickly respond to changing conditions, reducing delays in decision-making and improving overall efficiency. By leveraging live data streams, businesses can gain valuable insights that were previously difficult or impossible to obtain in real time, especially in dynamic environments such as manufacturing, logistics, and customer service.
The application of live data streams enhances decision-making by providing up-to-the-minute information on key metrics. This leads to more accurate predictions, faster responses to issues, and the ability to proactively adjust strategies. For example, a retail chain might monitor real-time sales data to adjust inventory levels or promotions dynamically, or a logistics company could track the real-time location and condition of shipments to optimize routes and delivery times.
Key Benefits of Live Data Streams in Decision-Making
- Faster Response Times: Immediate access to live data allows for faster identification and resolution of issues.
- Increased Accuracy: Continuous data flow provides more accurate insights, improving forecasting and resource allocation.
- Proactive Adjustments: Organizations can anticipate problems and make adjustments before issues escalate.
Real-Time Data in Various Sectors
Industry | Application | Impact |
---|---|---|
Manufacturing | Monitoring equipment and production processes | Minimized downtime, optimized maintenance schedules |
Retail | Real-time sales data for dynamic inventory management | Improved stock levels, reduced overstock/understock |
Logistics | Tracking shipments and optimizing delivery routes | Faster deliveries, lower operational costs |
Real-time analytics allow organizations to make operational decisions that were previously reactive, shifting to proactive and predictive strategies.