Statistical Power Analysis for the Behavioral Sciences 1977
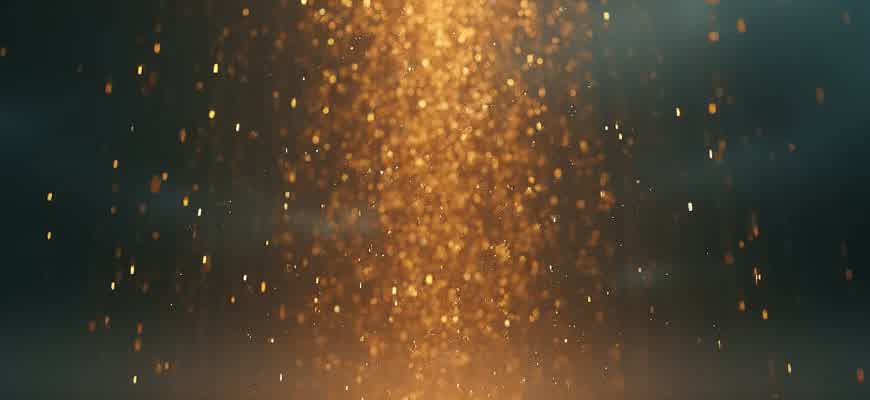
The concept of statistical power is crucial in the design and evaluation of psychological and behavioral research. It helps researchers assess the likelihood that a study will detect an effect if one truly exists. In 1977, a seminal work emerged, shedding light on the methodology and application of power analysis within the context of behavioral sciences. This framework has since become foundational in experimental psychology, offering tools to determine sample sizes and understand the potential of statistical tests.
Key Elements of Power Analysis:
- Effect Size: The magnitude of the difference or relationship being tested.
- Sample Size: The number of observations or subjects in the study.
- Significance Level (Alpha): The threshold for rejecting the null hypothesis.
- Statistical Test: The method used to analyze the data (e.g., t-tests, ANOVA).
Power Calculation: Core Formula
Variable | Description |
---|---|
Power | Probability of detecting a true effect, if one exists. |
Effect Size | Magnitude of the observed effect in the study. |
Sample Size | Number of participants or observations in the study. |
Alpha Level | Risk of committing a Type I error (false positive). |
"Power analysis not only helps in designing studies but also in interpreting their findings, ensuring that the study has a high probability of detecting meaningful effects." - Statistical Power Analysis for the Behavioral Sciences, 1977.
How "Statistical Power Analysis" Enhances Behavioral Research Precision
In the realm of behavioral sciences, making reliable inferences from experimental data is crucial. Statistical power analysis plays a key role in ensuring that the studies are not only designed efficiently but also provide meaningful results. This tool helps researchers determine the optimal sample size, ensuring that their experiments have a high probability of detecting actual effects. By focusing on both the sensitivity of a test and its capacity to avoid Type II errors (false negatives), power analysis directly influences the robustness and credibility of behavioral research findings.
Beyond sample size, power analysis offers a systematic approach to controlling experimental design variables. Researchers can manipulate different factors–such as effect size, sample size, and significance level–to understand their interactions and optimize research designs. With this analytical tool, researchers gain a clearer understanding of their data and the potential for uncovering genuine behavioral effects, leading to more precise conclusions.
Key Benefits of Statistical Power Analysis in Behavioral Research
- Improved Decision-Making: Researchers can determine if the sample size is adequate before conducting the experiment, minimizing the risk of underpowered studies.
- Reduced Risk of Type II Errors: Power analysis helps reduce the chance of failing to detect a real effect due to insufficient statistical power.
- Enhanced Resource Efficiency: It enables optimal use of resources, including time and participants, by avoiding over- or under-sampling.
Critical Factors in Power Analysis
- Effect Size: A measure of the magnitude of the relationship or difference being studied. Larger effect sizes typically require smaller sample sizes to detect.
- Sample Size: The number of participants in a study. Larger samples increase statistical power but also require more resources.
- Significance Level: Often denoted as alpha (α), this is the threshold for determining whether an observed effect is statistically significant.
"Statistical power analysis is the cornerstone for designing experiments that yield reliable and accurate insights in behavioral science, providing the clarity needed for informed conclusions." - 1977, Statistical Power Analysis for the Behavioral Sciences
Example of Power Analysis Impact
Effect Size | Sample Size | Power |
---|---|---|
Large | 30 | 0.85 |
Medium | 100 | 0.80 |
Small | 200 | 0.75 |
Applying Power Analysis to Design Robust Experiments in Psychology
Power analysis is a critical tool in the design of psychological experiments, providing a method to assess the likelihood that a study will detect an effect if it truly exists. By calculating the power of a statistical test, researchers can determine the sample size required to achieve reliable and meaningful results. This concept is rooted in statistical theory and has been widely adopted across various domains of psychology to ensure that experiments are neither underpowered nor wastefully overpowered.
One of the primary uses of power analysis is to balance the risk of Type I and Type II errors in experimental design. Researchers can optimize their experimental conditions and resources to avoid drawing incorrect conclusions from small or inadequately sized samples. This process also enhances the interpretability and validity of research findings by providing a clear foundation for understanding statistical results.
Key Steps in Applying Power Analysis
- Choosing the Statistical Test: Identify the appropriate statistical test based on the research question and experimental design (e.g., t-test, ANOVA, regression).
- Defining the Effect Size: Estimate the expected effect size, which represents the magnitude of the difference or relationship under investigation.
- Setting Alpha and Beta Levels: Determine the significance level (alpha, typically 0.05) and the power level (beta, typically 0.80) to minimize Type I and Type II errors.
- Calculating the Sample Size: Use software tools or statistical tables to calculate the minimum sample size required to achieve the desired power.
Power Analysis in Psychological Research
Power analysis also guides the interpretation of previous studies. If a study has low statistical power, the results might not be reliable or replicable, leading to potential issues with reproducibility in psychology. Therefore, understanding and applying power analysis can significantly improve the overall quality of psychological research.
Important Consideration: Adequate power reduces the likelihood of committing a Type II error, which is crucial when investigating small effect sizes or working with complex behavioral phenomena.
Example of Power Analysis Calculation
Parameter | Value |
---|---|
Effect Size (d) | 0.50 |
Alpha Level | 0.05 |
Desired Power | 0.80 |
Sample Size (per group) | 64 |
Calculating the Right Sample Size for Behavioral Studies Using Power Analysis
Sample size determination is a critical step in designing behavioral studies. It directly influences the accuracy and reliability of the conclusions drawn from the research. Power analysis provides a systematic approach to calculating the optimal sample size, ensuring that the study has enough participants to detect meaningful effects without being underpowered or excessively overpowered. Properly sized samples help to avoid issues like Type I (false positives) and Type II (false negatives) errors, which can skew results and reduce the generalizability of the findings.
Several factors contribute to the determination of the appropriate sample size in behavioral studies. These factors include the effect size, significance level, and the desired statistical power. Understanding the relationships between these variables allows researchers to design studies that are both efficient and effective. A sample size that is too small might fail to identify important effects, while a sample size that is too large might waste resources and increase the likelihood of finding statistically significant but practically irrelevant results.
Key Factors in Sample Size Calculation
- Effect Size: Represents the magnitude of the difference or relationship being studied. Larger effect sizes require smaller sample sizes to detect significant differences.
- Alpha Level (α): The probability of committing a Type I error, typically set at 0.05. This indicates the threshold for rejecting the null hypothesis.
- Power (1-β): The probability of correctly rejecting the null hypothesis when it is false, commonly set at 0.80 (80% power), meaning the study has an 80% chance of detecting an effect if it exists.
Steps to Calculate Sample Size
- Determine the effect size: Use previous research or pilot studies to estimate the expected effect size. Common benchmarks for effect size include small (0.2), medium (0.5), and large (0.8) for Cohen's d.
- Choose the desired power and significance level: Select a power level (usually 0.80) and an alpha level (usually 0.05).
- Use power analysis tools: Use statistical software or power analysis calculators to compute the sample size based on the chosen parameters.
Example Calculation Table
Effect Size | Alpha Level (α) | Power (1-β) | Required Sample Size |
---|---|---|---|
0.2 (Small) | 0.05 | 0.80 | 400 |
0.5 (Medium) | 0.05 | 0.80 | 64 |
0.8 (Large) | 0.05 | 0.80 | 26 |
In behavioral sciences, accurately determining the right sample size is fundamental to producing valid and reliable results. Underpowered studies run the risk of failing to detect real effects, while overly large samples can lead to inefficiencies in terms of time, resources, and interpretation of statistical significance.
Integrating Power Analysis into Statistical Software for Behavioral Science Projects
Power analysis is a crucial step in behavioral science research, allowing researchers to determine the likelihood of detecting true effects in their studies. By incorporating power analysis into statistical software, researchers can make informed decisions about sample sizes, effect sizes, and statistical tests, improving the reliability and validity of their findings. As statistical software tools evolve, integrating power analysis directly into these platforms streamlines the process, making it more accessible and efficient for users in the behavioral sciences.
When power analysis is integrated into software, it enables real-time feedback during study planning, which is especially beneficial for researchers with limited statistical expertise. Such integration not only helps with optimizing study design but also guides the choice of appropriate statistical tests based on the desired power level and sample size. This improves the overall quality of research outcomes and reduces the risk of Type I and Type II errors.
Key Benefits of Integration
- Improved Study Design: Researchers can adjust sample sizes and parameters on the fly, ensuring that the study is adequately powered to detect meaningful effects.
- Guided Decision-Making: Integrated tools provide recommendations on statistical tests, effect sizes, and power thresholds based on the research context.
- Reduced Errors: By optimizing power, the likelihood of both false positives (Type I errors) and false negatives (Type II errors) is minimized.
How Power Analysis Can Be Used in Behavioral Science Projects
- Sample Size Determination: Power analysis can suggest an optimal sample size that balances practical constraints and statistical rigor.
- Effect Size Estimation: Helps researchers estimate the minimum effect size that can be detected with a given sample size.
- Test Selection: Assists in selecting the most appropriate statistical test based on the study’s design and objectives.
“Integrating power analysis into statistical software enables a more accessible and transparent approach to study design, benefiting both novice and experienced researchers in the behavioral sciences.”
Example of Power Analysis in Behavioral Research
Parameter | Recommended Value |
---|---|
Desired Power | 0.80 or higher |
Alpha Level | 0.05 |
Effect Size | Medium (Cohen's d = 0.5) |
Sample Size | Depends on the desired power and effect size |
Understanding the Relationship Between Effect Size and Statistical Power
Effect size and statistical power are fundamental concepts in the field of behavioral science research, particularly when evaluating the results of hypothesis tests. Understanding the relationship between these two elements is crucial for designing experiments that have adequate sensitivity to detect meaningful differences or relationships. The effect size represents the magnitude of a phenomenon, while statistical power refers to the probability of correctly rejecting the null hypothesis when it is false. A higher effect size typically increases the power of a statistical test, meaning that the study is more likely to detect a true effect if one exists.
While both effect size and statistical power are related, they are not identical concepts. The power of a test depends not only on the size of the effect but also on other factors such as sample size and the chosen significance level (α). Researchers must balance these elements to ensure that their studies are not underpowered (too small to detect meaningful effects) or overly large (leading to unnecessary resource expenditure). Below is an exploration of this relationship through a more detailed breakdown of the key variables that influence both metrics.
Key Factors Influencing Effect Size and Statistical Power
- Sample Size: A larger sample size increases both the precision of the effect size estimate and the statistical power, making it easier to detect smaller effects.
- Significance Level (α): A higher α (e.g., 0.05 vs. 0.01) increases power, but it also increases the chance of a Type I error (false positive).
- Effect Size: As the effect size grows, so does the power of the test, provided that the sample size remains constant.
Effect Size and Power: A Practical Example
Effect Size | Power | Sample Size |
---|---|---|
Small | Low | Large |
Medium | Moderate | Moderate |
Large | High | Small |
"Effect size and statistical power are not just statistical concepts but are critical tools for designing research that maximizes efficiency while maintaining scientific rigor."
Minimizing the Risk of Type II Error
- Increase Sample Size: This is the most straightforward way to increase power.
- Choose a Larger α: Increasing the significance threshold slightly can reduce the likelihood of a Type II error but at the cost of potentially raising Type I error.
- Use a More Sensitive Test: Selecting the correct statistical method based on the data characteristics can improve power.
Identifying Common Pitfalls in Power Analysis for Social Science Research
Power analysis is a critical tool for designing research studies, particularly in the field of social sciences, where sample sizes and effect sizes often need careful consideration. Despite its importance, there are several common pitfalls that researchers may fall into when performing power analysis. These errors can lead to misleading results, wasted resources, and ultimately undermine the validity of findings. Below are some of the key mistakes frequently encountered in power analysis within social science research.
One of the primary issues researchers face is an insufficient understanding of how to correctly interpret power analysis results. Many rely too heavily on default settings or oversimplified assumptions that do not reflect the complexity of social science data. Recognizing and addressing these errors is crucial for obtaining reliable and meaningful outcomes. The following sections explore these pitfalls in greater detail.
Common Mistakes in Power Analysis
- Neglecting the Assumptions Behind Statistical Models: Researchers often overlook the assumptions that underpin their statistical models. These assumptions include normality, homogeneity of variance, and linearity. Failing to check these assumptions can result in a power analysis that doesn't accurately reflect the actual conditions of the study.
- Overestimating the Effect Size: Another frequent error is the overestimation of the expected effect size. This can lead to overly small sample sizes, which increases the risk of Type I and Type II errors. Accurate effect size estimation is essential for determining an appropriate sample size.
- Misinterpreting the Significance of Power: Some researchers confuse statistical power with the probability of obtaining a significant result. While power refers to the likelihood of detecting a true effect if one exists, it does not guarantee the discovery of significant findings, especially if the study is underpowered or poorly designed.
Key Areas to Focus On in Power Analysis
- Sample Size Determination: A critical aspect of power analysis is determining the appropriate sample size. Using too small a sample can lead to insufficient power, while too large a sample can be inefficient and wasteful.
- Effect Size and Its Estimation: Accurate estimation of the expected effect size is vital. Researchers should use previous studies or pilot data to estimate realistic effect sizes rather than relying on arbitrary assumptions.
- Type I and Type II Errors: It's essential to strike a balance between these errors. A high Type I error rate (false positives) can undermine the credibility of findings, while a high Type II error rate (false negatives) means missing important effects.
Power analysis should not be seen as a mere checklist item in research design. It requires careful consideration of study parameters, assumptions, and realistic expectations to avoid misleading conclusions.
Table: Power Analysis Pitfalls and Solutions
Pitfall | Potential Solution |
---|---|
Neglecting model assumptions | Conduct thorough diagnostic checks (e.g., normality, homogeneity of variance) before performing the analysis. |
Overestimating effect size | Base effect size estimates on prior research or pilot studies, and avoid overly optimistic assumptions. |
Misunderstanding power | Focus on the broader context of research design, including potential risks of underpowered studies and misinterpretation of significance. |