Conversion Table Z-score to Percentile
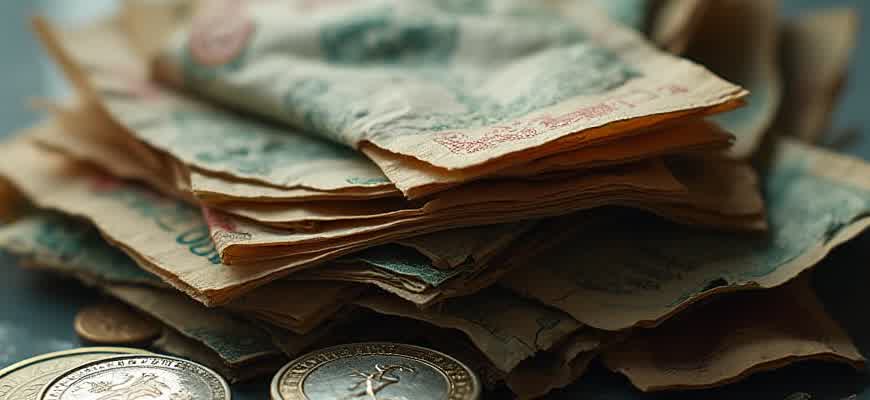
The Z-score is a standardized score that measures how far a data point is from the mean of a distribution, expressed in terms of standard deviations. Converting Z-scores into percentiles allows us to better understand the relative position of a data point within a dataset. This process is useful in various fields such as statistics, psychology, and finance for assessing probabilities and making decisions based on data.
To facilitate this conversion, a table is often used. Below is a simplified version of such a table, which shows the approximate percentile corresponding to a given Z-score. Keep in mind that Z-scores and their associated percentiles are typically derived from the cumulative distribution function (CDF) of the normal distribution.
Important: Z-scores of 0 represent the mean, with a corresponding percentile of 50%. Positive Z-scores indicate values above the mean, while negative Z-scores indicate values below it.
Z-Score to Percentile Conversion Table
Z-Score | Percentile |
---|---|
-2.00 | 2.28% |
-1.50 | 6.68% |
-1.00 | 15.87% |
0.00 | 50.00% |
1.00 | 84.13% |
1.50 | 93.32% |
2.00 | 97.72% |
Understanding the Z-Score and Its Role in Statistics
The Z-score, often referred to as the standard score, is a key statistical measure used to describe the position of a data point relative to the mean of a data set. It represents how many standard deviations a given data point is away from the mean. By converting raw scores into Z-scores, it becomes easier to compare data points from different distributions or scales. This transformation is particularly valuable when dealing with variables that are measured on different units or scales, allowing for an apples-to-apples comparison.
The Z-score plays an integral role in various statistical analyses, such as hypothesis testing and the calculation of percentiles. It serves as a standardized measure, making it possible to interpret data within the context of a normal distribution. A positive Z-score indicates that the data point is above the mean, while a negative Z-score shows that it lies below the mean. Understanding this concept is essential for interpreting data in a wide range of fields, from psychology to finance.
Key Features of the Z-Score
- Standardization: The Z-score standardizes data to make it comparable across different distributions.
- Unit-Free Measure: Z-scores are unit-less, meaning they don't rely on the units of measurement used for the original data.
- Interpretation: A Z-score allows you to understand how extreme a particular data point is in the context of the overall distribution.
How Z-Scores Are Calculated
- First, calculate the mean (average) and standard deviation of the data set.
- Next, subtract the mean from the data point of interest.
- Finally, divide this difference by the standard deviation.
Formula for Z-Score: Z = (X - μ) / σ
Where X is the data point, μ is the mean, and σ is the standard deviation of the data set.
Understanding Z-Scores and Percentiles
When examining a Z-score in the context of a normal distribution, the Z-score can be used to determine the percentile rank of a data point. This percentile tells you the percentage of data points that fall below the given value in the distribution. Z-scores are directly related to percentiles, which help in understanding the relative position of an individual data point.
Z-Score | Percentile Rank |
---|---|
0 | 50% |
1.0 | 84.13% |
-1.0 | 15.87% |
2.0 | 97.72% |
How to Convert Z-Scores to Percentiles with Simple Steps
Converting Z-scores to percentiles allows us to better understand the relative position of a value within a distribution. The Z-score represents the number of standard deviations a data point is from the mean, while percentiles indicate the percentage of data points that fall below a particular value. The process of conversion is straightforward when you have access to the Z-score table, also known as the standard normal distribution table, or when using online tools or statistical software.
To convert a Z-score to a percentile, follow a few simple steps. First, locate the Z-score in the standard normal distribution table. Then, identify the corresponding percentile value. The Z-score gives you the probability of a data point being less than the specific value, which directly translates to the percentile.
Step-by-Step Guide to Conversion
- Find the Z-score for which you need the percentile.
- Use a Z-score table to look up the cumulative probability associated with the Z-score.
- Convert this cumulative probability to a percentile by multiplying the probability by 100.
- The resulting value is the percentile corresponding to the given Z-score.
Important: Z-scores above 0 represent values greater than the mean, and Z-scores below 0 represent values less than the mean. The higher the Z-score, the higher the percentile.
Example Conversion
Z-Score | Percentile |
---|---|
1.00 | 84.13% |
-1.00 | 15.87% |
2.00 | 97.72% |
For example, a Z-score of 1.00 corresponds to the 84.13th percentile. This means the data point is higher than 84.13% of all other data points in the distribution.
Common Pitfalls in Understanding Z-Scores and Percentiles
Converting between Z-scores and percentiles is essential in statistics for understanding data distribution. However, this process can be tricky, and misinterpretations often occur, especially for those new to these concepts. Common mistakes arise when individuals overlook the relationship between the standard normal distribution and the actual dataset being analyzed, leading to confusion in how results are applied.
One of the main challenges is incorrectly assuming that a Z-score always corresponds to the same percentile across different distributions. In reality, Z-scores are tied to the standard normal distribution, and percentiles can vary depending on the specific dataset. Let’s explore some of the frequent mistakes made during this conversion.
1. Confusing Z-Scores with Percentiles
Z-scores represent the number of standard deviations a data point is from the mean, but they are not directly equivalent to percentiles. The percentile indicates the percentage of data points that lie below a certain value. However, a Z-score provides a position relative to the mean and does not directly show this percentage without conversion.
- Incorrectly assuming that a Z-score of 1.96 corresponds to the 95th percentile in all cases (it’s true only for the standard normal distribution).
- Failing to adjust the Z-score when dealing with non-normal distributions, which may lead to inaccurate percentile calculations.
2. Misunderstanding Tail Areas and Symmetry
Another mistake is neglecting the symmetrical nature of the normal distribution when interpreting percentiles associated with extreme Z-scores.
Important Note: Z-scores in the tails (e.g., +3 or -3) represent extreme values, but the corresponding percentiles should reflect the cumulative probability on either side of the mean.
- Assuming a Z-score of 2.0 always represents a cumulative percentile of 97.5%. This is true for standard normal distributions, but for other distributions, the corresponding percentile may differ.
- Not accounting for the fact that the lower and upper tails of the distribution are mirror images of each other in a standard normal curve.
3. Incorrect Use of Conversion Tables
Using Z-score to percentile conversion tables improperly can lead to miscalculations. It's important to remember that these tables assume a standard normal distribution. If the dataset doesn’t follow this distribution, applying such a table will yield misleading results.
Z-Score | Percentile |
---|---|
-2.0 | 2.28% |
0.0 | 50.00% |
2.0 | 97.72% |
When to Use a Z-Score to Percentile Conversion Table
Conversion tables for Z-scores to percentiles are essential tools when working with data that follows a normal distribution. Z-scores represent how many standard deviations a particular data point is from the mean. However, Z-scores alone do not provide direct insight into the relative standing of a value within a distribution. A conversion table allows you to map a Z-score to its corresponding percentile, helping to interpret the position of data points more effectively.
These tables are particularly useful in statistical analysis, research, and quality control, where understanding the relative position of a value in a normal distribution is crucial. For example, if you need to understand how a specific score compares to the overall population, a Z-score to percentile table gives you a quick, standardized way to make that comparison.
When Should You Refer to the Conversion Table?
Here are some common scenarios where a conversion table for Z-scores is highly valuable:
- Determining Percentile Ranks: When you have a Z-score and want to determine the corresponding percentile of that value within a population.
- Evaluating Performance: In academic or psychological testing, when you need to interpret the performance of an individual relative to others in a normal distribution.
- Data Analysis and Research: When working with data sets that follow a normal distribution and you need to standardize results for comparison purposes.
Key Considerations
The Z-score to percentile conversion assumes that your data approximates a normal distribution. If the data is skewed or follows a different distribution, using such a table could lead to inaccurate results.
Example Table
Z-Score | Percentile |
---|---|
-2.0 | 2.28% |
0.0 | 50.00% |
2.0 | 97.72% |
As shown in the table, a Z-score of -2.0 corresponds to the 2.28th percentile, indicating that the value is below 98% of the data points in a standard normal distribution. This insight can be invaluable when interpreting results in research or assessments.
Real-World Applications of Z-Score to Percentile Conversion
Understanding the relative position of a data point within a distribution is crucial for making informed decisions in many fields. By converting a Z-score to its corresponding percentile, professionals can translate abstract statistical concepts into actionable insights. This conversion is particularly valuable in fields such as education, healthcare, and finance, where understanding how individual scores or values relate to a broader group is essential.
For example, when interpreting test scores or medical measurements, knowing the percentile rank helps assess whether a given value is typical, unusually high, or low compared to the general population. Below are several real-world applications where this conversion plays a key role:
Key Applications
- Education: In standardized testing, converting Z-scores to percentiles allows educators and students to evaluate how a student’s performance compares to that of peers, providing valuable context for assessing achievement.
- Healthcare: In medical research and diagnostics, Z-scores are used to compare test results (e.g., cholesterol or blood pressure) to the average population. Converting these to percentiles helps doctors understand whether a patient’s result is within a healthy range.
- Finance: Investors and analysts use Z-scores to evaluate stock price volatility or risk, and converting these to percentiles provides a clearer understanding of how a specific asset behaves relative to the market.
Example of Application in Education
Consider a standardized exam where a student receives a Z-score of 1.5. This Z-score corresponds to a percentile rank of approximately 93.32%, indicating that the student performed better than roughly 93% of test-takers. This information helps in making decisions about further education or scholarship eligibility.
Z-Score | Percentile |
---|---|
1.5 | 93.32% |
-0.5 | 30.85% |
0.0 | 50.00% |
When Z-scores are converted to percentiles, they allow for easier interpretation in real-world contexts, especially when comparing individual data points to a wider group. This is particularly useful in education, healthcare, and finance.
How Reliable Are Z-Score to Percentile Conversion Tables?
When using Z-score to percentile conversion tables, it is important to recognize their limitations. These tables provide a quick way to estimate the percentile corresponding to a given Z-score, but they come with a degree of approximation. The accuracy of these tables is highly dependent on the method used to generate the Z-scores and the assumptions underlying the data distribution. Z-scores assume a normal distribution of data, but real-world data may deviate from this ideal, which can affect the precision of the corresponding percentiles.
While Z-score to percentile conversion tables are useful for rapid calculations, they may not always reflect the exact percentile for non-normally distributed datasets. Tables are usually rounded, and slight inaccuracies in values can accumulate when applied in large datasets or critical analyses. Despite this, for most practical applications, these tables provide a sufficient level of accuracy for general purposes.
Factors Affecting the Accuracy
- Distribution Assumptions: Z-scores assume a normal distribution, but real-world data may be skewed or follow a different distribution.
- Rounding Errors: Most conversion tables round Z-scores to a few decimal places, which may lead to small discrepancies when calculating exact percentiles.
- Sample Size: In large datasets, small inaccuracies in conversion tables can accumulate and have a more significant impact on results.
Common Usage and Limitations
- Quick Estimation: These tables are typically used for rapid, approximate calculations, such as in educational contexts or when quick decision-making is required.
- Approximation of Percentiles: While they provide an estimated percentile, the exact value might be slightly off, especially for extreme Z-scores.
- Limited to Normal Distribution: Tables are designed for normally distributed data and might not be applicable to datasets with skewed or non-symmetric distributions.
The more skewed the data, the less reliable the Z-score to percentile conversion will be, as the normality assumption no longer holds true.
Example of Conversion
Z-Score | Percentile |
---|---|
1.00 | 84.13% |
1.96 | 97.5% |
2.58 | 99.5% |
How to Interpret Percentiles in the Context of Your Data
Percentiles offer valuable insights when analyzing the distribution of data. They provide a way to understand the relative standing of a specific data point within a dataset. By converting raw scores into percentiles, you can interpret how a particular value compares to the rest of the dataset in terms of position. This can be especially helpful for identifying trends, patterns, and anomalies within the data.
For example, if a student scores at the 90th percentile in a standardized test, it means they performed better than 90% of the test-takers. Understanding percentiles allows you to assess whether a value is high, low, or typical compared to the rest of the data, helping you make more informed decisions based on relative comparisons.
Understanding the Role of Percentiles
- Percentiles help in assessing where a data point stands within a broader distribution.
- They allow comparison between data points from different datasets or groups, even if they have different scales or units.
- By identifying the percentile rank, you can determine whether the value is exceptional, average, or below expectations.
Important: A percentile rank indicates the percentage of scores below a certain value. It does not tell you anything about how far apart those scores are.
Key Percentile Ranges
- Lower Percentiles (1st to 25th percentile): Data points in this range are on the lower end of the distribution.
- Middle Percentiles (25th to 75th percentile): Values in this range represent the central portion of the dataset, indicating typical or average values.
- Higher Percentiles (75th to 99th percentile): Data points in this range are higher than the majority of values in the dataset.
Example of a Percentile Table
Percentile Rank | Interpretation |
---|---|
10th | Data point is better than 10% of the values. |
50th | Data point is at the median, better than 50% of the values. |
90th | Data point is better than 90% of the values. |
Why You Need a Z-Score to Percentile Conversion Tool Online
When working with statistical data, converting a Z-score to a percentile is often crucial for understanding the relative position of a data point within a distribution. Z-scores are useful for standardizing values, but they don’t provide a direct interpretation in terms of percentage rank. A Z-score to percentile converter tool simplifies this process by quickly transforming the Z-score into a more intuitive percentile value. This is particularly beneficial for professionals in education, psychology, and research who require precise, fast results.
Rather than manually consulting Z-score tables or performing complex calculations, using an online tool ensures accuracy and saves time. With the right tool, you can instantly calculate percentiles for various distributions, even when working with non-standard data. This is particularly valuable when analyzing data sets that deviate from typical Gaussian distributions, as the tool can easily adjust to different parameters.
Advantages of Using an Online Conversion Tool
- Speed: Converts Z-scores to percentiles in seconds, eliminating the need for manual calculations.
- Accuracy: Provides precise percentile values, reducing human error in statistical analysis.
- Convenience: Available anytime and anywhere, perfect for students, researchers, and professionals on the go.
- Customizability: Some tools allow you to input different distribution parameters, making them adaptable to various scenarios.
How It Works: A Quick Guide
- Enter the Z-score value into the conversion tool.
- The tool applies the appropriate formula to calculate the corresponding percentile.
- Instantly receive the percentile, ready for analysis or reporting.
Important: Ensure that the Z-score you input matches the specific distribution assumptions for accurate percentile conversion.
Example Conversion
Z-Score | Percentile |
---|---|
1.0 | 84.13% |
-1.0 | 15.87% |
0.0 | 50% |