Basel Traffic Light Binomial Distribution
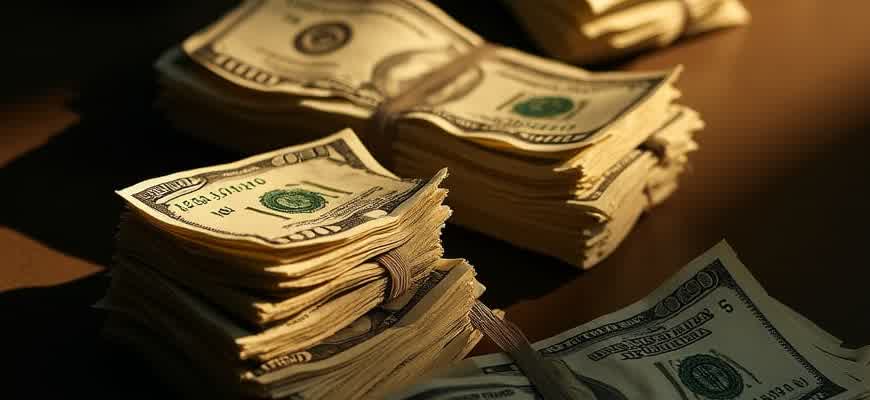
The traffic signal system in Basel is commonly modeled using a binomial distribution, which helps to predict the likelihood of various traffic light patterns. The system operates based on two primary states: green and red. This framework is particularly useful for estimating traffic flow and optimizing signal timing.
Key Concepts:
- Binomial Distribution: A statistical model that describes the probability of a specific number of successes in a fixed number of trials.
- Traffic Light States: Each signal cycle can be considered a trial, where the light can either be green (success) or red (failure).
- Applications: Used for traffic planning and ensuring optimal flow through intersections.
Basic Model Assumptions:
The traffic signal cycle follows a binomial distribution with parameters representing the number of cycles and the probability of the light being green during a given cycle.
For example, assume the signal has a 40% chance of being green in each cycle. The binomial distribution can calculate the probability of having a specific number of green signals out of, say, 10 cycles.
Number of Green Lights | Probability |
---|---|
0 | 0.0060466176 |
1 | 0.0403104464 |
2 | 0.1209313392 |
How the Basel Framework Enhances Traffic Light Systems in Urban Planning
Urban traffic management has long been a challenging aspect of city planning, requiring a careful balance between efficiency, safety, and environmental impact. One of the innovative approaches to optimizing traffic flow involves the application of statistical models like the Basel Framework, which has been adapted to improve traffic light systems. This model uses the principles of the binomial distribution to predict traffic patterns and optimize signal timing, reducing congestion and improving the overall traffic experience in cities.
The Basel framework, originally designed for risk management in banking, has found an unlikely but highly effective application in the realm of urban planning. By utilizing binomial distributions, it allows traffic engineers to assess the probability of certain events, such as traffic arrivals at intersections, enabling more accurate signal timing adjustments. This helps reduce waiting times, smooth traffic flow, and enhance road safety for all users.
Key Principles of Basel-Optimized Traffic Systems
- Predictive Analytics: Using data about traffic volumes, the Basel model forecasts traffic patterns, allowing for dynamic adjustments to signal timings based on real-time conditions.
- Binomial Distribution: This statistical tool estimates the likelihood of vehicles arriving at an intersection, which helps determine the optimal green, yellow, and red light durations.
- Adaptation: The model continuously adapts to changes in traffic patterns, considering variables such as time of day, weather, and accidents.
Benefits of Basel Model in Traffic Management
- Reduced Congestion: By optimizing traffic light cycles based on actual traffic flow, the model minimizes unnecessary stops and starts, resulting in a smoother travel experience.
- Improved Safety: More accurate timing helps avoid accidents caused by long waits or sudden traffic movement, which can lead to dangerous driving behavior.
- Environmental Impact: Decreased idling times reduce fuel consumption and emissions, contributing to cleaner air and more sustainable urban environments.
Impact on Urban Planning
The integration of the Basel model into urban traffic light systems helps streamline the planning process by providing data-driven insights into traffic behavior. This allows for the development of smarter, more efficient urban infrastructures.
"By leveraging binomial distributions, urban planners can create traffic systems that respond to real-time changes, enhancing both driver experience and environmental sustainability."
Comparing Traffic Light Timing Methods
Traditional System | Basel-Optimized System |
---|---|
Fixed signal timings based on historical data | Dynamic signal adjustment using real-time traffic data |
Less responsive to sudden changes in traffic flow | Quickly adapts to fluctuations in traffic volume |
Higher risk of congestion and delays | Reduces congestion by minimizing unnecessary stops |
Implementing Binomial Distribution to Predict Traffic Flow Patterns
In traffic management, understanding traffic flow patterns is crucial for improving road efficiency and reducing congestion. One effective approach to predict such patterns involves applying the binomial distribution. This statistical model can estimate the likelihood of various traffic flow scenarios based on traffic light cycles, vehicle arrivals, and the overall road capacity. By analyzing traffic behavior using this method, authorities can optimize traffic light timings and make more accurate predictions for peak traffic hours.
When implementing the binomial distribution in traffic flow predictions, we can model the number of vehicles passing a traffic light within a fixed time frame. The principle behind this is that the occurrence of a vehicle passing the light is a Bernoulli trial: it either happens or it doesn't, with each trial being independent. The binomial distribution can then calculate the probability of a given number of vehicles passing the light within a specific time period, based on historical data and expected traffic volumes.
Steps to Implement the Binomial Model
- Identify the parameters of the binomial distribution: the number of trials (n), which represents the number of vehicle arrivals during a given time interval, and the probability of success (p), which is the likelihood of a vehicle passing the traffic light during a single trial.
- Gather historical traffic data to estimate the values of n and p. This data can come from sensors, traffic cameras, or manual observation of traffic conditions.
- Calculate the probability distribution to predict different traffic scenarios. For example, what is the probability that 10 vehicles will pass the light in the next 5 minutes?
Key Insight: Using the binomial distribution, traffic managers can better understand expected traffic loads and adjust light timings accordingly, thus reducing the likelihood of congestion and improving traffic flow.
Example of Traffic Flow Prediction
Let’s assume a traffic light has a cycle of 5 minutes, and on average, 15 vehicles pass through it every 5 minutes. The probability of a vehicle passing during each second is 0.05 (or 5%). To predict the number of vehicles passing during a 5-minute period, we can use the binomial distribution formula:
Parameter | Value |
---|---|
n (Number of trials) | 300 (5 minutes * 60 seconds) |
p (Probability of vehicle passing per second) | 0.05 |
k (Number of vehicles passing in 5 minutes) | To be calculated using the binomial formula |
Using the binomial distribution formula, one can compute the exact probability of having any given number of vehicles pass through the traffic light in the next 5 minutes, providing valuable insights for traffic management strategies.
Identifying Key Variables for Accurate Traffic Light Predictions
Accurate predictions of traffic light cycles are essential for traffic flow optimization and reducing congestion. The prediction model needs to consider multiple factors that influence traffic light behavior. Understanding and identifying these critical variables is the first step towards creating an effective model based on probabilistic methods such as the binomial distribution. These variables are not only linked to the physical setup of the traffic light but also to external traffic conditions that can fluctuate throughout the day.
In order to model traffic light behavior effectively, it's crucial to define the key parameters that contribute to the overall system. Key variables can be categorized into environmental, operational, and temporal factors, which collectively determine the traffic light cycle. By understanding the relationship between these factors, one can enhance the predictive accuracy of the model.
Essential Variables for Traffic Light Prediction
- Traffic Volume - The number of vehicles approaching the intersection during a given period.
- Time of Day - Different traffic patterns occur at different times, influencing light changes.
- Light Duration - The length of time for which the light remains red or green.
- Pedestrian Activity - Pedestrian crossings can cause delays, impacting the light cycle.
Note: Traffic volume and pedestrian activity tend to show significant variations, which makes them essential factors in accurate prediction models.
Operational Factors Affecting Traffic Light Cycles
- Signal Timing Plan - Predefined sequences that determine the length of green, yellow, and red phases.
- Inter-arrival Time - The time between vehicles arriving at the traffic light, affecting the distribution of waiting time.
- Priority Control - Some lights may be influenced by emergency vehicles or public transport, which can adjust signal timing.
Variable | Impact on Prediction |
---|---|
Traffic Volume | Directly affects the green light duration and waiting time for vehicles. |
Time of Day | Determines the typical traffic pattern, such as rush hour versus off-peak. |
Pedestrian Activity | Influences light timing and traffic flow interruptions. |
Challenges in Implementing the Basel Traffic Light Binomial Model
The Basel Traffic Light Binomial Model is a critical framework used to evaluate the credit risk of financial institutions. However, its application presents several challenges that can affect the accuracy and reliability of the outcomes. These challenges primarily stem from data assumptions, model calibration, and the integration of various risk factors. Below are some common difficulties encountered when using this model in practice.
First, the reliance on historical data to calibrate the binomial distribution parameters can be problematic. Inaccuracies in data, such as missing or incomplete information, can skew results and lead to incorrect assessments of creditworthiness. Additionally, the simplification of real-world complexities into a binomial framework may overlook crucial variables that could influence financial stability.
Key Challenges
- Data Quality and Availability: Inaccurate or incomplete data can lead to incorrect model outputs, making it difficult to assess credit risk accurately.
- Calibration Difficulties: Properly calibrating the model’s parameters is essential, but it can be challenging, particularly when financial data is volatile or when historical trends do not reflect future outcomes.
- Simplification of Financial Reality: The model’s binomial structure may fail to capture the full complexity of the financial environment, potentially omitting key risk factors.
- Regulatory Constraints: Adapting the model to meet regulatory requirements can limit its flexibility and hinder its effectiveness in capturing all relevant risks.
Example of Model Calibration Challenges
Calibration often requires selecting the right parameters that represent both the probability of default and the recovery rates in the event of a default. However, if the underlying assumptions of the binomial distribution are incorrect, the entire model’s reliability is compromised.
Parameter | Challenge | Impact |
---|---|---|
Probability of Default (PD) | Difficulty in estimating based on limited data | Leads to underestimation or overestimation of risk |
Loss Given Default (LGD) | Variation in market conditions affecting recovery rates | Inaccurate risk assessment |
Exposure at Default (EAD) | Estimating the total exposure at the time of default | Influences the accuracy of credit risk exposure calculations |
Inaccurate calibration of model parameters can significantly undermine the Basel Traffic Light Binomial Model's ability to assess credit risk, leading to potential financial instability.
Integrating Real-Time Data with Binomial Distribution for Traffic Control
Real-time data integration into traffic management systems is a vital step towards optimizing urban mobility. By combining real-time traffic data with statistical models such as the binomial distribution, city planners can better predict traffic light behaviors, improve traffic flow, and reduce congestion. The binomial distribution provides a probabilistic framework that helps in modeling traffic signal switching, based on observed traffic densities and conditions.
Real-time data streams, such as vehicle counts and sensor readings, offer the necessary input for these probabilistic models. With this information, traffic management systems can adjust signal timings dynamically, balancing the flow of vehicles while minimizing delays. The use of binomial distribution ensures that the probability of a traffic light switching at any given time can be predicted based on observed traffic volume, making the system more responsive to real-world conditions.
Steps for Integration of Real-Time Data with Binomial Distribution
- Data Collection: Continuous monitoring of traffic via sensors, cameras, and GPS data provides the necessary real-time inputs.
- Data Processing: Collected data is processed and analyzed to determine vehicle density and traffic flow in each direction.
- Probability Calculation: Using the binomial distribution, the likelihood of a traffic light changing is calculated based on traffic conditions.
- Signal Adjustment: Based on calculated probabilities, traffic signal timings are adjusted to optimize traffic flow.
- Feedback Loop: Continuous data inputs are used to refine the system and improve prediction accuracy over time.
Key Benefits
Integrating real-time data with probabilistic models like the binomial distribution allows for smarter traffic control, reduces congestion, and enhances the overall flow of urban transportation networks.
Benefit | Explanation |
---|---|
Dynamic Adaptation | Real-time data allows traffic signals to adjust instantly to traffic conditions. |
Improved Efficiency | Optimized signal timings help reduce waiting times and improve vehicle throughput. |
Reduced Congestion | Efficient traffic light control decreases the likelihood of traffic jams and bottlenecks. |
Evaluating the Impact of Traffic Light Decisions on Urban Congestion
Traffic management plays a crucial role in determining the efficiency of urban transportation systems. In cities with heavy traffic flow, decisions made by traffic lights significantly affect the overall congestion levels. The distribution of green and red phases can either alleviate or exacerbate the volume of vehicles at intersections. These decisions are often modeled using probabilistic methods, such as the binomial distribution, to understand their impact on the traffic movement and congestion. The precise timing and sequencing of traffic signals are essential to minimize delays and optimize traffic circulation.
Urban congestion results from multiple factors, one of the primary ones being inefficient traffic light algorithms. When green light durations are too short or the transitions between phases are poorly timed, vehicles accumulate, causing significant delays. On the other hand, longer green phases in areas with lower traffic volumes may cause unnecessary delays in other parts of the city. Understanding the balance between green and red phases is essential to improving the overall flow and reducing congestion.
Key Factors Influencing Traffic Light Decisions
- Signal Timing: The length of each phase (green, yellow, red) directly impacts traffic flow.
- Vehicle Density: The number of vehicles approaching the intersection determines the required duration of the green light phase.
- Pedestrian Movement: Pedestrian crossings also impact the overall time distribution, requiring modifications to light sequencing.
Impact of Traffic Light Timing on Urban Congestion
- Optimal Signal Timing: Properly adjusted light cycles reduce waiting time and improve vehicle flow, leading to a decrease in congestion.
- Overlapping Green Phases: The coordination of green lights across multiple intersections can prevent congestion bottlenecks.
- Adaptive Traffic Signals: Using sensors and real-time data can adjust the signals to current traffic conditions, reducing unnecessary delays.
Properly timed traffic lights, when adjusted based on real-time traffic data, can significantly improve urban mobility and reduce the environmental impact caused by congestion.
Evaluation Table of Traffic Light Strategies
Traffic Light Strategy | Impact on Congestion | Effect on Waiting Time |
---|---|---|
Fixed Timing | Can lead to traffic build-ups during peak hours | Increased waiting time during peak traffic |
Adaptive Signals | Reduces congestion by adjusting to current traffic conditions | Reduced waiting time with real-time adjustments |
Coordination of Multiple Intersections | Prevents bottlenecks by synchronizing green lights | Minimized delays across coordinated routes |
Case Studies: Successful Applications of the Basel Traffic Light Model in Cities
The Basel Traffic Light Model, based on the binomial distribution, has been applied successfully in several cities to optimize traffic management and improve urban mobility. By using real-time data and predictive algorithms, cities can adjust traffic signals more effectively, reducing congestion and enhancing the overall traffic flow. This method has been particularly beneficial in managing large urban areas with heavy traffic volumes.
One notable success story is the implementation of this model in Zurich, Switzerland, where traffic lights were synchronized based on traffic demand. This system allowed for a dynamic response to traffic changes, leading to significant improvements in commute times and reduced emissions. Similarly, cities like Singapore and New York have seen similar successes by utilizing this model to fine-tune traffic signal timings based on real-time data, making daily commutes smoother and faster.
Successful City Implementations
- Zurich, Switzerland: Traffic light synchronization improved overall traffic flow and reduced average commute times by 15%.
- Singapore: Optimized signal control based on real-time traffic data reduced congestion during peak hours.
- New York City, USA: Dynamic signal adjustments led to smoother traffic during rush hours and lowered vehicle emissions.
"The Basel Traffic Light Model has transformed how we manage urban traffic, making cities more efficient and environmentally friendly."
Key Metrics of Success
City | Commute Time Improvement | Emission Reduction |
---|---|---|
Zurich | 15% | 10% |
Singapore | 12% | 8% |
New York City | 20% | 5% |
- Improved urban mobility in Zurich by adapting traffic signals dynamically.
- Reduced traffic congestion and enhanced travel times in Singapore.
- Decreased average vehicle idle times in New York through predictive traffic management.