Intelligent Traffic Systems Examples
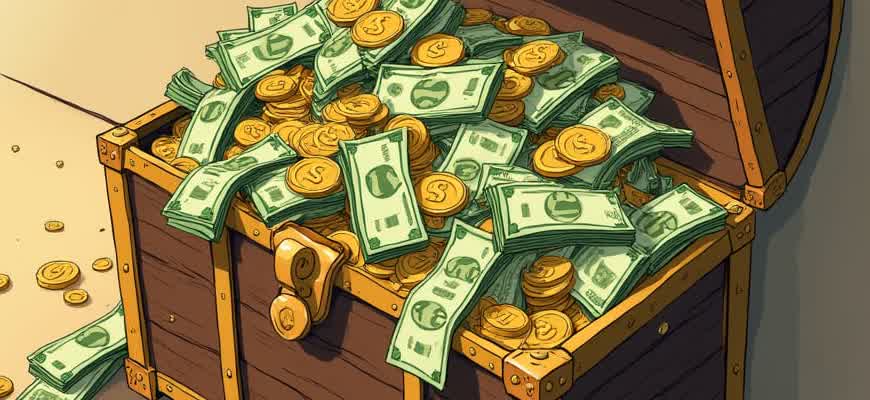
Intelligent transportation systems (ITS) are designed to improve traffic flow, reduce congestion, and enhance safety through the use of technology. These systems integrate real-time data, advanced algorithms, and communication technologies to optimize traffic control and management.
Here are some common examples of ITS in use today:
- Adaptive Traffic Signal Control: Adjusts traffic light timings based on real-time traffic conditions.
- Automatic Number Plate Recognition (ANPR): Used for monitoring vehicles and enforcing traffic laws.
- Dynamic Message Signs (DMS): Provides real-time traffic updates, such as accidents or road closures.
- Vehicle-to-Infrastructure (V2I) Communication: Allows vehicles to communicate with traffic signals to optimize travel times.
Example of Adaptive Traffic Signal Control:
"Adaptive traffic signals are designed to respond to real-time traffic patterns. For example, if there is a heavy flow of vehicles on one street, the system adjusts the signal timings to give more green time to that road, reducing congestion and improving overall traffic movement."
Another example of ITS includes the use of sensors and cameras embedded in roadways to detect and analyze traffic volumes. These systems enable traffic operators to manage the flow of vehicles in real time, adjusting for peak hours or special events.
Technological Components:
Technology | Description |
---|---|
Radar Sensors | Detect vehicle speed and movement. |
Inductive Loop Sensors | Embedded in roads to detect the presence of vehicles. |
Video Analytics | Uses cameras and AI to monitor and analyze traffic patterns. |
How Smart Traffic Lights Optimize Urban Traffic Flow
Smart traffic signals are a key component in modern intelligent transportation systems, designed to reduce congestion and improve the efficiency of traffic flow in urban areas. These systems use real-time data, sensors, and advanced algorithms to dynamically adjust the timing of traffic lights based on traffic conditions. The integration of technologies such as machine learning and big data allows these systems to adapt to varying traffic patterns, reducing wait times and enhancing overall road network performance.
By responding to the current flow of traffic, these systems enable smoother transitions through intersections, particularly during peak hours. The ability to prioritize traffic movement, such as giving more green time to roads with higher volumes, helps optimize the distribution of vehicles, minimizing delays and preventing bottlenecks. Additionally, smart traffic lights can interact with other elements of the urban transport infrastructure, like pedestrian crossings and public transportation, to create a seamless experience for all users of the road.
Key Features of Smart Traffic Lights
- Adaptive Signal Control: Adjusts the signal timing in real-time based on traffic flow.
- Vehicle Detection: Uses sensors or cameras to detect the number and type of vehicles at intersections.
- Data-Driven Decision Making: Utilizes historical and real-time traffic data to predict and optimize signal timing.
- Integration with Other Systems: Works alongside public transport and pedestrian signals for better traffic management.
Benefits
- Reduced waiting time for vehicles and pedestrians.
- Lower fuel consumption and emissions due to reduced idling times.
- Decreased congestion, particularly during rush hours.
- Improved safety through better traffic management and smoother transitions at intersections.
Traffic Signal Optimization Example
Feature | Description |
---|---|
Adaptive Timing | Adjusts the duration of green and red lights based on real-time traffic data. |
Emergency Vehicle Prioritization | Allows emergency vehicles to pass through intersections more quickly by automatically extending green lights. |
Pedestrian Detection | Detects pedestrian presence and adjusts signal timing to allow safer crossings. |
"Smart traffic lights do not only optimize the flow of vehicles, but also contribute to creating safer, more sustainable urban environments by reducing delays and improving the predictability of traffic patterns."
Real-World Benefits of Adaptive Signal Control in Smart Cities
Adaptive signal control is a key component of intelligent traffic systems that dynamically adjust traffic light timings based on real-time traffic conditions. This technology provides significant improvements in traffic flow, safety, and environmental impact, making it an essential tool for smart cities. By using real-time data from sensors, cameras, and GPS, these systems can optimize the timing of traffic lights to minimize congestion and reduce wait times.
In smart cities, where the goal is to increase efficiency and sustainability, adaptive signal control systems offer tangible benefits. These systems not only enhance the overall driving experience but also contribute to reducing energy consumption and emissions, making urban transportation greener. As more cities implement such solutions, the long-term positive impact on traffic management becomes increasingly evident.
Key Advantages of Adaptive Traffic Light Systems
- Reduced Congestion: By adjusting signal timings in real-time, adaptive systems minimize traffic bottlenecks and keep vehicles moving more smoothly.
- Improved Safety: These systems can prioritize emergency vehicles, reducing response times and improving public safety.
- Lower Environmental Impact: By reducing idle time and preventing unnecessary stops, emissions are decreased, helping to improve air quality.
- Cost Savings: Cities can reduce the need for additional infrastructure or road expansions, making adaptive control systems a cost-effective solution for urban planning.
How Adaptive Traffic Systems Work
- Data Collection: Sensors and cameras gather real-time traffic data from various locations in the city.
- Signal Adjustment: Based on the data, the system adjusts signal timings dynamically, optimizing traffic flow.
- Continuous Monitoring: The system continuously assesses traffic conditions to adapt to changing patterns throughout the day.
"By continuously adjusting traffic lights based on real-time data, adaptive signal control systems not only improve traffic flow but also contribute to the overall reduction of congestion and environmental harm."
Impact on Traffic Flow Efficiency
Traffic Condition | Traditional Traffic Signals | Adaptive Signal Control |
---|---|---|
Heavy Traffic | Long wait times, congestion | Reduced wait times, better flow |
Low Traffic | Unnecessary stops | Optimized signal times |
Emergency Vehicles | Delays, blocked routes | Prioritization of emergency response |
Case Study: Successful Implementation of AI-Driven Traffic Management
Artificial Intelligence (AI) has proven to be a game-changer in traffic management, offering innovative solutions to optimize urban mobility. One of the most successful implementations of AI in this field has been seen in the city of Barcelona, Spain. The city integrated AI-powered systems into its traffic infrastructure to reduce congestion, enhance road safety, and improve overall traffic flow. By leveraging AI algorithms and real-time data from traffic cameras, sensors, and GPS devices, Barcelona achieved remarkable improvements in traffic management.
The AI system in Barcelona uses advanced machine learning models to predict traffic patterns and adjust traffic signal timings in real-time. This dynamic approach allows the city to respond to changing traffic conditions instantly, ensuring smoother movement of vehicles and reducing the likelihood of gridlocks. The AI-driven system has become a benchmark for other cities seeking to implement smart traffic solutions.
Key Benefits of AI-Driven Traffic Systems
- Reduced Traffic Congestion: AI systems optimize signal timings to minimize traffic buildup during peak hours.
- Improved Road Safety: The system helps monitor traffic violations and prevent accidents by detecting hazardous driving behaviors.
- Real-Time Traffic Monitoring: Sensors and cameras continuously provide data, allowing the system to make immediate adjustments.
Implementation Process
- Data Collection: AI systems gather data from traffic sensors, cameras, and GPS devices across the city.
- Analysis: Machine learning algorithms process the data to predict traffic trends and identify patterns in real time.
- Signal Adjustment: Based on the analysis, traffic lights and road signage are adjusted dynamically to optimize vehicle flow.
Results
"Since the implementation of AI-driven traffic management, Barcelona has seen a significant decrease in average travel times, a reduction in fuel consumption, and an overall improvement in road safety."
Metric | Before AI | After AI |
---|---|---|
Average Travel Time | 30 minutes | 20 minutes |
Fuel Consumption | High | Reduced by 15% |
Accidents | Moderate | Decreased by 10% |
Real-Time Traffic Monitoring Using IoT Technology
The integration of Internet of Things (IoT) technology into traffic management systems has revolutionized the ability to monitor and manage road conditions in real-time. By utilizing a network of connected sensors, traffic cameras, and communication devices, cities can gather detailed information about traffic flow, congestion, and road safety, allowing for timely adjustments to traffic signals and more effective route planning. This interconnected approach enhances the overall efficiency of transportation networks and contributes to reducing traffic delays, fuel consumption, and environmental impact.
IoT-driven traffic monitoring systems enable the collection of vast amounts of data, which can then be analyzed for decision-making purposes. This process helps city planners and authorities identify problem areas, predict traffic patterns, and optimize the use of infrastructure. Below are key components of IoT-based traffic monitoring solutions:
- Traffic Sensors: Installed on roads, bridges, and intersections to track vehicle speed, volume, and type.
- Connected Cameras: Use image recognition and AI to identify traffic incidents and congestion in real-time.
- Communication Systems: Enable the transfer of traffic data to central hubs for processing and analysis.
- Smart Traffic Lights: Automatically adjust based on traffic flow data received from sensors.
Real-time data monitoring improves decision-making and can be leveraged for various purposes such as dynamic traffic signal adjustments, predictive maintenance, and the creation of more efficient routing systems. The table below outlines the advantages of using IoT for traffic monitoring:
Benefit | Description |
---|---|
Improved Traffic Flow | IoT systems dynamically adjust traffic lights based on live data, minimizing congestion. |
Incident Detection | Instant notifications when accidents or roadblocks are detected, facilitating quick responses. |
Energy Efficiency | Reduced traffic idling leads to lower fuel consumption and a decrease in emissions. |
Predictive Maintenance | IoT-enabled monitoring systems can predict infrastructure wear and tear, allowing for proactive maintenance. |
Real-time IoT monitoring can dramatically reduce the time spent in traffic, improving overall urban mobility and contributing to more sustainable cities.
How Smart Traffic Systems Improve Public Safety and Reduce Accidents
Smart traffic management systems play a crucial role in enhancing road safety by optimizing traffic flow and minimizing human errors. Through the use of real-time data collection, these systems monitor vehicle speeds, traffic density, and road conditions. By making adjustments based on this data, they prevent dangerous situations before they occur. Smart systems can provide early warnings of hazards, like congestion or accidents, and redirect traffic to safer routes, ultimately reducing the likelihood of collisions.
These systems also improve emergency response times by prioritizing traffic signals for emergency vehicles. By reducing delays, they ensure that paramedics, fire trucks, and police can reach accident sites more quickly, thus increasing the chances of saving lives. Furthermore, intelligent traffic solutions include predictive algorithms that detect potential accident-prone areas, offering preventive measures such as speed limits adjustments or automatic traffic light changes.
Key Features Contributing to Safety Enhancement
- Adaptive Signal Control: Traffic lights adjust based on real-time traffic conditions to minimize congestion and reduce the chance of accidents.
- Vehicle-to-Infrastructure Communication: Vehicles can communicate with traffic signals to avoid collisions and improve overall traffic flow.
- Incident Detection: Automated systems can detect accidents or traffic irregularities, triggering immediate responses like sending alerts to drivers and authorities.
Types of Accidents Reduced by Smart Traffic Systems
- Rear-End Collisions: Adaptive signal control reduces stop-and-go traffic, which is a common cause of rear-end crashes.
- Intersection Accidents: Real-time monitoring and automated light adjustments can prevent accidents at intersections caused by driver error or traffic mismanagement.
- Pedestrian Incidents: Pedestrian crossings are monitored, and vehicles are alerted to reduce speeds when pedestrians are detected nearby.
"By reducing congestion and optimizing signal timings, smart traffic systems contribute significantly to safer roads and fewer accidents, offering long-term benefits for public safety."
Example: Smart Traffic Implementation in a Major City
Feature | Impact |
---|---|
Real-time Traffic Data Collection | Helps adjust signal timings to manage congestion and reduce accidents. |
Emergency Vehicle Prioritization | Improves emergency response time and ensures quicker access to accident sites. |
Accident Detection Algorithms | Identifies incidents and notifies authorities immediately for rapid response. |
The Role of Big Data in Predicting Traffic Patterns and Congestion
Big data plays a crucial role in understanding and forecasting traffic flow by processing massive amounts of real-time data. Traffic monitoring systems leverage a wide range of sensors, cameras, GPS devices, and social media feeds to collect relevant information. These data sources allow for precise traffic pattern analysis, which in turn aids in predicting congestion and optimizing traffic management systems. By analyzing historical and real-time traffic data, cities can develop accurate models to forecast peak traffic times, road usage, and potential bottlenecks.
Advanced algorithms and machine learning techniques are applied to detect patterns and predict traffic conditions. By integrating these technologies with intelligent traffic systems, cities can manage traffic more efficiently, reducing congestion and improving overall transportation infrastructure. Big data tools also help identify problem areas and suggest improvements, enabling proactive management and real-time interventions to reduce delays.
Key Data Sources and Predictive Tools
- Real-time traffic sensors
- GPS data from vehicles
- Traffic cameras
- Weather data
- Social media insights (user-generated content about traffic conditions)
How Big Data Helps in Predicting Congestion
- Historical Traffic Analysis: Analyzing past traffic data allows for the identification of recurring patterns, such as daily or seasonal peaks, and facilitates more accurate predictions.
- Real-time Data Integration: Data from various sources can be processed in real-time to offer dynamic predictions of road conditions and suggest alternate routes to reduce delays.
- Weather and Event Correlation: By factoring in external variables like weather conditions or large public events, big data systems can predict how these factors may exacerbate congestion.
Big data systems not only forecast congestion but also recommend real-time actions, such as adjusting traffic signal timings or rerouting traffic, to alleviate congestion.
Example of Predictive Analytics in Traffic Management
Data Source | Purpose |
---|---|
GPS Data | Track vehicle locations and estimate travel times across different routes. |
Traffic Cameras | Provide real-time visual feedback of road conditions, traffic volume, and accidents. |
Weather Data | Anticipate the impact of weather on traffic flow (e.g., rain, snow, fog). |
Integrating Autonomous Vehicles into Intelligent Traffic Networks
Integrating self-driving cars into modern traffic management systems poses significant challenges and opportunities. Autonomous vehicles (AVs) rely heavily on data exchange, sensors, and advanced algorithms to navigate and interact with their surroundings. As cities transition to smarter traffic networks, it becomes essential to ensure that AVs seamlessly interact with conventional vehicles, traffic signals, and infrastructure. The integration process involves updating communication protocols and adapting the system's infrastructure to support both autonomous and non-autonomous vehicles.
In order to achieve this, traffic networks must be equipped with technologies that allow for real-time data sharing, vehicle-to-vehicle (V2V), and vehicle-to-infrastructure (V2I) communication. This collaboration ensures efficient traffic flow, reduces congestion, and improves overall safety. Moreover, incorporating AVs into intelligent traffic systems also requires adjustments to road design, traffic signal patterns, and the development of specialized lanes for autonomous cars.
Key Challenges and Solutions
- Data Synchronization: Ensuring that all vehicles, including autonomous ones, have access to synchronized traffic information in real-time.
- Traffic Signal Coordination: Adjusting signal timings to accommodate autonomous vehicles' response times and predictability.
- Infrastructure Adaptation: Updating roads, signage, and other infrastructure elements to ensure compatibility with AV technology.
Approach to Integration
- Deployment of Communication Systems: Installing V2V and V2I communication systems to enable seamless interaction between all vehicles and traffic infrastructure.
- Data Sharing Platforms: Creating centralized platforms that collect and distribute real-time data on traffic conditions, accidents, and roadwork.
- Continuous Monitoring and Adjustment: Implementing adaptive traffic management systems that can adjust traffic flows dynamically based on AV behavior.
"The future of traffic management will depend on how effectively autonomous vehicles can be integrated into the existing network of roadways and infrastructure, allowing for a safer and more efficient travel experience."
Examples of Successful Integration
City | Technology Used | Outcome |
---|---|---|
San Francisco | Smart Traffic Lights, V2X Communication | Improved traffic flow and reduced accidents with autonomous vehicles on the roads. |
Singapore | Connected Infrastructure, AV Lanes | Enhanced coordination between autonomous vehicles and existing traffic systems, leading to smoother traffic patterns. |
Cost-Benefit Evaluation: Investing in Smart Traffic Management Solutions
Implementing intelligent transportation systems (ITS) requires a careful evaluation of both the costs involved and the potential benefits. The upfront investment in modernizing traffic infrastructure is significant, but the long-term advantages make it an attractive option for many cities and municipalities. This analysis provides insights into how smart traffic solutions can deliver both economic and operational benefits, enhancing the overall transportation experience.
Before making any decisions, it is essential to consider both tangible and intangible factors that contribute to the success of these systems. Smart traffic management can help reduce traffic congestion, improve road safety, and reduce environmental impact, making it a valuable investment. However, proper cost assessment and ROI (Return on Investment) evaluation are crucial for determining the project's viability.
Key Cost Factors
- Initial Setup Costs: Includes the purchase and installation of sensors, cameras, and communication networks.
- Maintenance and Upgrades: Ongoing costs for software updates, hardware repairs, and system monitoring.
- Training and Workforce: Training personnel to operate and maintain the new system can be a significant expense.
Benefits of Smart Traffic Solutions
- Reduced Traffic Congestion: Traffic management algorithms optimize flow, decreasing wait times and reducing overall congestion.
- Improved Road Safety: Real-time traffic monitoring helps detect accidents and hazards, reducing the likelihood of collisions.
- Environmental Impact: Reduced emissions due to smoother traffic flow and decreased idling time of vehicles.
"Smart traffic systems can save billions annually by improving traffic flow and reducing accidents, with benefits far exceeding initial implementation costs."
Cost-Benefit Comparison
Investment Category | Estimated Cost | Long-Term Benefit |
---|---|---|
Initial Setup | $10 million | Improved traffic flow, less congestion |
Maintenance | $500,000/year | Better traffic management, real-time response to incidents |
Safety Enhancements | $200,000 | Reduced accidents and fatalities, improved public safety |